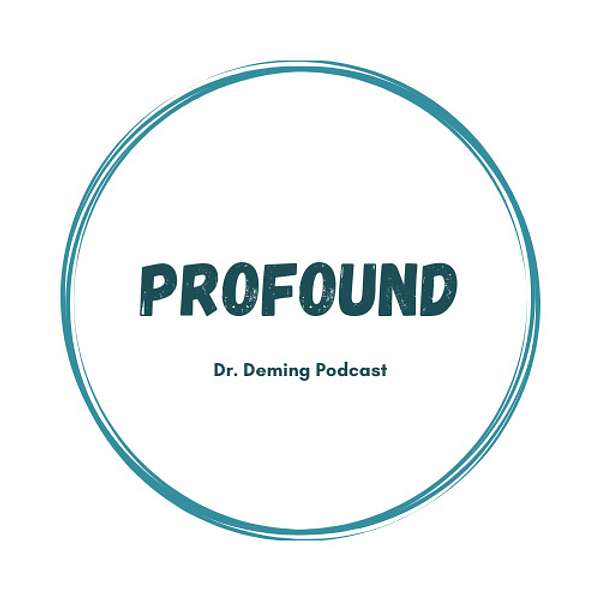
Profound
Ramblings about W. Edwards Deming in the digital transformation era. The general idea of the podcast is derived from Dr. Demming's seminal work described in his New Economics book - System of Profound Knowledge ( SoPK ). We'll try and get a mix of interviews from IT, Healthcare, and Manufacturing with the goal of aligning these ideas with Digital Transformation possibilities. Everything related to Dr. Deming's ideas is on the table (e.g., Goldratt, C.I. Lewis, Ohno, Shingo, Lean, Agile, and DevOps).
Profound
S3 E8 - Bill Bellows - Genichi Taguchi and Quality - Part 1
In this first part of a two-part series, I chat with Bill Bellows in this episode. Bill gave us a little history of his introduction to Genichi Taguchi's methods and quality concepts while working as an engineer in the jet engine industry. We explore key ideas around variability reduction, precision vs accuracy, process capability, and Taguchi's loss function. Our conversation looks at the difference between traditional quality approaches focused on specifications and Taguchi's view of quality based on quantifying loss and variation. We riff on analogies using a dartboard and Deming's red bead experiment to clarify Taguchi's perspectives on "loss" and variation even within specification limits. Bill's LinkedIn can be found here:
https://www.linkedin.com/in/bill-bellows-218435/
John Willis: [00:00:00] Hey, this is John Willis, another episode of Profound. I got a really cool guest now. I've been fortunate since the book came out to start meeting a few more people. In the traditional Deming world and just some fascinating people and here's one of them. I just I'm gonna play up a little bit bill but like there's there's certain people when they talk you feel like you have to take notes faster and they can talk but I've been fascinated by everything I've heard from bill and I thought it was a It'll be awesome for y'all to get the, to hear Bill and meet Bill.
And what we're going to try to do here is a multi series cause I think limiting his knowledge to one podcast would be a crime in my opinion. So we're going to cover and we're going to, we're going to sort of dance around some really cool topics. But let me start off Bill. Why don't you go ahead and introduce yourself?
Bill Bellows: Yeah. Bill Billows, mechanical engineer by background spent. A lot of time in graduate school, spent nine years in college, and then went to [00:01:00] work in the jet engine business for seven years in the East Coast. And then in my last few years there, I got exposed to the work of Genichi Taguchi and Deming and some others and decided it was where I wanted to spend the rest of my career.
My original career was a heat transfer engineer on jet engines, and that was my, my PhD research was. Was in that same field so I did that for seven years and or so I then decided this this Deming Taguchi stuff is I just thought it was a lot more exciting. And so I spent a couple of years looking and turned out a company here in Los Angeles was looking for someone with my background and I just jumped at the opportunity with a new daughter who was born in that timeframe and headed West and worked there for 26 years at Rocketdyne and then left there in 2016 for a position as deputy director.
With the Deming [00:02:00] Institute where I reported to Kevin Cahill and I had been on the board of the Deming Institute for about 12 years. So I was, as a board member, I was aware of what they did, which is much different than being an employee. I used to kid Kevin, when I was an outsider, Kevin Cahill, you know, who was the president of the Deming Institute was my, was my was my boss.
And, and I would say, you know, I'd say, Hey, Kevin, if you ever need any help, just, you know, just let me know. And until I went to work that I didn't realize when you're on the inside. You need a lot more help than I could offer. And so as a part of what was fascinating is to find out what goes on and then realize, boy, outsiders can only do so much.
Cause these are some really full time. Needs and and so part of my role is responding to inquiries when people wanted to use a quote from Dr. Deming or photograph of Dr. Deming or they had interest in learning more, did Dr. Deming's ideas apply to nonprofits, for [00:03:00] example, and I say, why do you ask? And I'd say, well, most of what I read about Dr.
Deming seems to apply only to manufacturing. And so it looks like it only applies to manufacturing and I'd say, no, no, he worked with companies that did manufacturing, but it's bigger than that. So those are the types of inquiries I would respond to. And, and it was just, but the inside, it was just a fascinating 2 years and 8 months.
And then I decided to go off and I was restricted in that role. I wanted to do teaching, do a lot more teaching, so now I do teaching for online courses for Southern Utah University and Cal State Northridge. And those things I just could not do working with the Deming Institute. There was just no time for that.
And I also consult, and the Deming Institute doesn't do consulting, so I, I just, it was time to move on and go do what I really, really love to do is, is, is, you know, facilitate learning. Whether it's through university courses [00:04:00] or working with. You know, with corporate clients, that's what I do. Very cool.
Yeah. I
John Willis: think, you know, I think surely like through this couple of series of podcasts, we can deep dive into sort of Deming and why, you know, why we're fascinated and why we think it's like the why Deming and the answer. But the thing I've been fascinated by you is the way you talk about what you learned from Taguchi and the way you talk about Russell Acoff.
So I thought maybe we'd start with this one with Taguchi and I think I heard one of your podcasts about how you first got introduced to Taguchi and then, so Why is he important? Like, let's assume some of our readers know the basics of profound knowledge and theory of variation. So, like, how'd you get introduced Taguchi and, like, what do we need to understand about Taguchi and why does it overlay Deming
Bill Bellows: so well?
Well, one thing I'll say is I met Dr. Deming twice. I met him in 1990. He spoke at Western Connecticut State University. And if people go online or Google and do it, I [00:05:00] think on YouTube, you can find A lecture that Dr. Deming gave in February of 1990 at Western Connecticut State University. And it's a lecture I was given like the VHS tape for that I posted and then people made some copies of it.
So there's, and if people can't find, they can reach out to me and I'll, I'll send them a link. So, so I saw him speak three times that day, never asked him a question. I don't know if I had questions. I was, it was, he was talking about things I'd never heard before. And then I attended in 1993. I attended his very last four day seminar, which was held.
Here in Los Angeles, there are about 500 people in the room, and I didn't ask a, I mean, that was a situation, John, where I could have asked questions, but I didn't ask questions because he was literally near death. He died 10 days after the seminar ended. He was wheeled in in a wheelchair. On oxygen because the prostate cancer had metastasized to his lungs.
The nurse would wheel him up on [00:06:00] stage. He would lecture for an hour. Not if that, and then go back to his room and we're assisting him. So I never asked him a question. And he was incredible too,
John Willis: right? Because that idea that like, he just felt there was so much more to teach. People would ask him like, why are you doing this?
And he was like,
Bill Bellows: well, sitting, sitting to my right, I remember sitting in the same seat, you know, wherever I was, but I know sitting to my right for four days was a rabbi and he was quietly praying for Dr. Deming every day, all day, four days. And so he was, he was to my right and a couple of days in during a break, he got the attention of one of the assistants of the company that.
Organize these conferences for docket that man, the conferences were run by a company called a Q. E. S. Quality enhancement seminars founded by a woman Deming bet back in the sixties, Nancy, our man who wrote a book called keys to [00:07:00] excellence, which they used to hand out in the four day seminars, and I would encourage our viewers to go to Amazon and look for keys to excellence.
Remember once, John, I was I was reading it on an airplane and I I think I got up to go get something and go to the bathroom and I came back and the stewardess saw the book when I picked it up, but she said, have you found them? So Nancy man who met Deming back in the 60s, when Deming became well known, she worked a deal with Deming that she would promote, sponsor, do all the things for his four day seminars, except get up on stage.
So she had a full time staff doing this. And so the rabbi got the attention of one of Nancy's assistants. Who got Nancy and then it's a Nancy's to my left. I don't know if I met her at that point. I later got to meet her and found out she used to work at rocket time. So she was a coworker literally of friends of, of, of my [00:08:00] coworkers back in the 60s.
So, so Nancy's to my left and the rabbi's to my right. And I'm sat in there and the rabbi starts to scold Nancy. I can't believe what you're doing to this man. Look at what you're doing to this man. Wow. And she turns to him and says, you have no idea. She said, she said, you have no idea that there's no place he'd rather be than where he is.
That's something. And, and, and she knew that he wanted. So, so anyway, I say that because I've been mentored by people that work very closely with Dr. Deming, who spent countless hours with Dr. Deming. I wasn't one of them, but I spent. Countless hours with Genichi Taguchi and countless hours with Russell Aikoff.
And, and I think I likely spent more time with the two of them than anyone in the Deming community. And I knew as I was meeting them, getting to know them, that what they were having is somewhat [00:09:00] known in the Deming community, but not, I mean, not to the degree that I was getting from them with Dr. So I met Dr.
Taguchi in 1987. 87 or 88, something like that. I heard about him while working in Connecticut. I taken some training in problem solving, decision making, and then got involved in being a trainer of this material, Keppner Trago problem solving, decision making, and they, the number one product we made there was the, a 1500 horsepower engine.
For the gas turbine engine for the army's main battle tank. And the company was making about 120 engines a month, all told 10, 000 were made. And having started, I started there in 80s, 83 with my PhD. And now I was doing my thing, doing what they were hiring me for. He transferred research and development on the, for jet engines.
And, and, but I, [00:10:00] I eventually began to realize. That there would be the sounds in production on different engines. We made it a variety of engines for helicopters and small business jets and things like that. And then they'd be these task forces and a task force is it is it East Coast activity where.
There's a big problem. We're going to go throw all these bodies at it and go fix it trivia contest on the West Coast. There are not task forces. They're actually what's called Tiger teams. And then in Salt Lake City, where I consulted, they're called FABs. Which stands for failure analysis board, success analysis board.
So these are teams of people that come together ad hoc to go dive in and get us out of this rut. And, and so these, these things happen. And I was never involved in that activity. It was going around in the periphery, but I, well, then once I became a certified instructor in this problem solving decision making, part of it was [00:11:00] that I would be the engineering delegate to go help those teams that involved engineering people.
And so there was a, a. A very high visibility problem in the tank engine that had affected that then impacted all the engines that were already sold, which were quite a few. And a lot of these engines were in tanks in Europe, Western Europe, as the front line of defense in the Cold War. So it was a, and the issue that came up happened within, within 50 hours of use of these engines.
And the concern of the generals was that every one of those tanks. Maybe defective and what good does that do if the Soviets, you know, come in the Western Europe. So there's a lot of visibility, right? And so people are running engines and test cells, 24, for months. And then once a month, somebody had to go to the army and give them up, give them all these progress reports and the progress reports, you know, we're talking to [00:12:00] the world's best people in the area of the failure, which happened to be some gears war through each other.
And so if you look, if you think of gears having teeth and the teeth from one turning the teeth from the other, what happened was the teeth wore through each other, so there was nothing being trans, there's no torque being transmitted. And, and so months and months goes by, goes by. And finally, one of these status meetings that the army was hosting, one of them said to our delegate, have, have you folks ever heard of this thing called Taguchi methods?
And our person said no. And they said, tell you what? It says. The transmission people at General Motors, whenever they have problems like this, they use this Taguchi Methods thing. So, you take the action to send somebody to General Motors. And we'll take the action to let them know you're coming. And I, and I, so I was at this meeting where that was being explained.
You know, so the guy who went to the meeting and got that action came back and [00:13:00] said, you know, we got the action to send somebody to Indianapolis. And I, and I turned to the program manager at the tank engine. His name was Harold. I said, so Harold, who's going to go to Indianapolis? He says, you are, you are.
And I said, why me? He said, cause you're the problem solving guy. And what is so funny? I didn't, I didn't think I was the one to go. I was like, whoa. Why me? And he said, well, you're the problem solver I got, which fit. And so I did some research online, you know, we had a, you know, before the internet and I got some stuff back, which was obtuse.
I remember looking, I think I, I couldn't make heads or tails of it. So I went, and this was
John Willis: stuff about Taguchi
Bill Bellows: online. Yeah. Yeah. Yeah. And, but what's fascinating is I, I, I just didn't want to go and I went and I met their two Taguchi experts found out that they were trained. By what would have been one of GM's top Taguchi experts, not that I [00:14:00] knew anything that what this Taguchi stuff was, but what, what got my, the first thing that got my attention is that their top Taguchi expert had recently been reassigned from this transmission division to GM's Saturn Corporation and, and Tennessee, I think, and that got, that got my attention thinking, so you're, you're a guy person is, and I, is Saturn just, I guess, was big enough for me to constant, you know, to realize the thing and that, that sounds serious.
There's something about that just, just got my, really got my attention, but most of what I learned that day had nothing to do with very, very little to do with what I later realized is a real essence of Taguchi's work. What I got exposed to that day was. Fractional factorial experimentation, where, where instead of changing one factor, watching the output, changing another factor, watching the output, you change many [00:15:00] factors at one time in a, in a round of experiments.
And then I never, I think. All through graduate school, I went back and look, there was such courses in the catalog. But it wasn't on my radar screen or anybody that was mentoring me and my, my, my advisors. And so I walked away excited by this thing called a design of experiments and fractional factorial testing.
The, and that was part of the solution. A bigger part of the solution is that when I explained to them, they said, These two Taguchi guys said, so I saw in the hardware and all, he says, what do you measure? How do you run a test? I said, well, somebody has an idea as to what's causing these gears to wear, and we go run a test.
It's a 10 hour test that's designed to, you know, really cause these gears to wear. They, they, in an accelerated way. And he said, you know, how do you run the test? So we take a new set of gears, we put it in, and then we, and then we look at them. And he said, well, what measurements take [00:16:00] it? When he said that, I said.
The guy just, I'll show you what the parts look like. Oh, wow. This doesn't happen that often, John. It's not. So here is one part wine cup, because if I turn it up, it looks like a wine. And this is called the splined coupling. Ah, and the splined coupling has teeth on the outside and the wine cup has teeth on the inside.
Right. And so what would happen is, I remember being in one of these meetings, you know, trying to solve this thing. You know, somebody walks in direct from an engine test. It gives these two parts to the manager of the gear group, and the manager just holds them up to the light. That doesn't look Warren, that doesn't look Warren.
He just eyeballed it. So I said that to these guys in Indianapolis, I said, he just looks at them. And I had no reason to be suspicious of it. I'm not a gear guy. The guy looks, that's what they do. These are professionals. He says, it's black and white. He's treating it as [00:17:00] it's Warren or it's not. Yeah. He said, if you actually measure each tooth on each gear before and after, you're going to find there's a little bit of wear.
He says, you're throwing away a whole lot of information. He said, one is, you know, you're going to design the experiment changing more than one factor at a time. Two is, measure each gear before and after, measure every single tooth before and after, and how much material is worn. He says, it's not that it's worn or not worn, there's degrees of wear.
And then we're asking, well, how do you measure these things? And so there's a device called an optical comparator, you create a big shadow and you, you know, which is like a hundred times scale and you go, I said, I said, do we have one of those? He says, trust me. He says, you've got one of those devices.
Trust me. You're, you got one of those. So we went back and thought about a bunch of recent design changes that were made, seven different design changes. And we looked at, you know, each factor was a design change. And the way we looked at the variable, we said. [00:18:00] Let's look at the design before and after each change and ran a bunch of tests, ran eight tests actually nine tests and found out that one of those factors.
Was overwhelmingly causing the issue. It was a recent design change. So it was a combination of moving from a black and white looks good. Doesn't look good to shades of gray and, and fractional factorial experimentation. So I thought at that point in time, I probably thought fractional factorial experimentation, that's Taguchi.
Now it's, I mean, and that, and that hooked me on Taguchi, even though Taguchi is much more than that. So I did this. Yeah,
John Willis: well, you know, what's fascinating to like, in some ways, you know, at some point, I'd like to sort of pick your brain about how do we translate this to software delivery and all, but I think we're not there yet.
But in some ways, I listen at starting like, that's, and it's easy [00:19:00] to like, in a postmortem or, you know, Monday morning quarterback say. Duh, you know, like, would you like, like being making these engines and like the stress test is some person physically looking at it versus sort of measuring the changes, the before and after changes.
Right. And it just seems like, I mean, we were like, was that something that like Japan was just doing it? Like if we talk about like, I know we're too far down the sort of decimation of Japanese technology, the American technology, but just sounds like we were sort of. In a terrible rut from a quality perspective compared to like things like you're you go back.
Here's a, you know, I don't know at that point a billion dollar industry and, you know, and this is the way it's working. And you go to some people who GM who have picked up some of these fine tune ideas about quality. And then all of a sudden, there's a moment. I'm wondering how much in American quality.
Was more of the [00:20:00] black and
Bill Bellows: white. Well, a couple things come to mind. I would say just a postmortem. I, I don't know how usual what I saw was. I, I have no doubt that if we were measuring every tooth before and after, that might've been different. Now, what I'm part of is I'm hesitating is I, I don't know if the design change we discovered as the issue was examined prior to that test.
And, and, and that factor. Was a, a major contributor. So I don't, I, it's just that, and, but there are a couple of things you said that I'm going to go back to. So, so it may well be that they didn't look at that factor. And that was part of the reason that I couldn't see anything more so than black and white.
Perhaps so, so, but there's no doubt it was a combination of the block and shifting to measuring each tooth degree of where and, and that [00:21:00] factor being in there. Because if that factor is not on the table, then you're not going to find in that screening process anyway, to pick up on 1 thing you did say, I have no doubt in my mind that that organization's quality system was looking at everything, all new parts going into an engine.
As being good or bad that that, you know, a bearing is good or bad as not that I've measured bearings, but typically, you know, what I'm used to is there's a, an outer diameter that meets requirements and ID that meets requirements. Yeah, I'm used to maybe a surface roughness that meets requirements, you know, angles that meet requirements.
It was like a go, go, go, go, like, yeah, yeah, so I have, I have no doubt that the quality system that organization was going to and, but this issue was caused not by that quality system was caused by [00:22:00] a, a design change that was intended to improve how the oil flowed around those gears. So these, these two gears were in a, well, these two gears came together, they came together in a vertical passage, and so oil was flowing around them, and the design change that was made was intended to improve the oil flow around them.
So if you think of these two parts were, you know, let's say inside of a channel that might have been two inches in diameter. So this, this is about an inch in diameter. So these two parts are in the center and then there's a inside of a duct, which is let's say two inches and there's oil flowing around it.
So the design change that we found was instrumental in creating this problem was associated with people trying to improve the oil flow, which they did, but improving the oil flow screwed up the [00:23:00] lubrication of that interface. So that's not so much. You know how we look at quality, but but it got me exposed to quality and Taguchi's.
But I do recall that we're having this conversation. I remember reading Taguchi's explanation of quality in one of those documents that I got, and I'm looking at that thinking, what? What does that mean? And it was quality is the minimum of loss imparted to society by a product after it's shipped into the customer.
And I looked at that, what does that mean? So you want me to go to Indianapolis to learn about that? So in hindsight, as I'm thinking back, I'm thinking, so that was part of the reason I wasn't, I was thinking this is kind of a wild goose chases is how does lost society tie it? I don't know how this fits.
You know, nowadays, you know, I, I look at that statement and thinking, wow, but I, I was a different person and it just didn't make any sense to me.
John Willis: So, yeah, but what, so what is the, like the, all right, so it's, [00:24:00] it's obviously much more, you know, so the Taguchi loss function. And again, I'm, I'm like, I'm, I'm going out of my bounds to even use those expressions, but the, there was much more than just measuring before and after, right?
There is.
Bill Bellows: No, they you know, what I shared was that whole, that failure, you know, problem of the gear where it little did I realize how life changing that whole thing was that, I mean, I had already committed to being the problem solving facilitator, you know, for engineering. So I did problem solving training for the division.
So maybe once a month, I, I would co present a one week seminar. I signed up for that. I also signed up. If engineering had an issue, I would facilitate how they did decisions or solve problems, which was, which was exciting, but little did I see that this trip to [00:25:00] Indianapolis was going to be so life changing that I would, you know, first get sucked in to this fractional factorial design of experiments thing that I never heard about before, and we used it to solve this.
And, and then what happened next was the next time there was a big issue, I was called in to help solve it because one is, so now the company's thinking this Taguchi stuff solves problems. So when the next big issue came up, let's call Bill. And, and I was, again, everything I was doing was this fractional factorial, looking at number of factors thing and all the loss function thing until I got to Rocketdyne.
The last function thing didn't, that was way in the back burner. It was like, and I'm already thinking it was like very tangential. Now to me, that's, that's the, that's the entryway. I use his last function concept as the entryway, not as a tangential thing. Even though [00:26:00] I have all my conversations with Taguchi, he said to me, I was looking for, I said, can you, has anyone ever written, I said to him about how their organization matures.
In their use of the loss function, you know, from one, somebody comes back from taking a seminar and there's some textbook example and how does the organization, you know, take that and then refine that and then refine that and that's what I was looking for is, has anybody written about that and he says, no one in the United States uses the loss function.
So no one in the United States, he says, and then somehow he said, The leading users in the world are Toyota and Nippon Denso, now known as Denso, which is a major supplier to Toyota. They were originally
John Willis: Toyota, but in my research,
Bill Bellows: it was kind of interesting. Yeah. Yeah. There's there. I even think Toyota is a part owner of Denso, but when he said that, I thought I knew at that point that Toyota, I had not [00:27:00] yet purchased a Toyota, but that still meant something to me that, that.
That Toyota. Oh, no, no. When he made that statement, I had that first Toyota product my wife and I bought was an 89. And this conversation with Taguchi was 95, 96. So I already knew then that Toyota, there was something about Toyota. I knew they had Denso parts. So when he said they're the two leading users, I thought, wow, that, that explains something.
This is
John Willis: 1986 ish, you're saying? Not to today, this is the part where people are just starting to wake up to figure out, like, why are they beating the crap out of us?
Bill Bellows: Oh, that, that's, that's early 80s. Right, okay. I mean, Ford, in a, in a recent podcast for the institute, I think number 12 or 13, 11, number 11 has been posted, last night we recorded number 14.
But the Larry Sullivan, who used to be a senior manager in, I think for supply chain. [00:28:00] He wrote an article for Quality Progress, 19 80, 19 83 or 84, I think 83. And the article's entitled Variability Reduction on New Approach to Quality. So for those who are a SQ members, they can go online and find the article.
I'm pretty sure. You can get it with X. You can find it if you're a member. So the article, the article came out in 83. I've read it many times. And the article starts, starts in March of 1982. It says, I led a study mission to Japan to visit a number of suppliers, blah, blah, blah, blah, blah, blah. And the, the cover page of this article, 60 percent of the page, Is Dr.
Zaguchi's parabolic loss function, so a parabola, you think of it for our listeners as a bell shape. In this case, it's facing up, so on the cover is something like that with some Gaussian distribution super, and then, so 40 percent of the page, the bottom is text, you [00:29:00] know, so it's a Three columns. And so, you know, March of 82, I did this.
And on the right hand side, it says, you know, what we discovered in these visits is that Ford is that these supplier, these companies have a different approach to quality, much different. And it talks about how they focus on monitoring variability from targets. And all of that is on the front page. Right now, on the 2nd page, they missed the whole boat Sullivan missed the boat, which is a common experience.
But that article came out and what's significant is that article come out in 83. The visit was 82. So this is, you know, in 1982 is, I would say is likely the 1st time Ford went to Japan to go see what they're doing. So they were beginning to see an impact in market share. They knew, I mean, Ford. I mean, summer of 1980, Thon Peterson, part of his interest in As CEO of Ford [00:30:00] and reaching out to Deming is that they knew they had trouble.
It wasn't until 82 that Sullivan was able to assess that Taguchi's work was part. Of a differentiation, and so I would never proclaim that, you know, that that was the differentiator that was amongst the things, but that's interesting, though,
John Willis: that, like, that he points out, he sees it and then Taguchi in the 90s tells you, no, no American cut the only 2 companies.
He knows that at that point are still are using. His last function, right? There's there's interesting in that disconnect, right? Well, not it's because people just got overwhelmed with Deming sort of what they thought was reduction of variation and that like you said, the 2nd page of that report got it all wrong, right?
Is it that they really didn't understand Taguchi's work?
Bill Bellows: Well, I think it's really fascinating is that how many years later most people don't don't understand what to get you is talking about either [00:31:00] today. So, so, so, and to be fair, we probably get you to explain the last function. Let me say, let me say, let me offer to two things for this conversation.
Because like I said, we'll have, we'll have other, other opportunities to go further. But what I'd like to include in this episode is what I came up with about a year ago, working on a project for a client, and they're asking for some feedback. And I put in there, there's two fundamental, two fundamental questions of quality management.
And then the first question is, does this characteristic meet requirements? You know, does this diameter meet requirements? Does this flow rate meet requirements? Does this thickness meet requirements? Does this strength meet requirements? So there's a, you know, it's a good, you would call it a quality characteristics.
So a dimension, a size, does it meet requirements? And there's only two answers to that question. Yes or no. That's the black and white thinking that [00:32:00] I first got exposed to in Indianapolis. So John, most, I'm not aware of any organization in the world that doesn't define quality operationally without question number one, does this meet requirements?
So that's simplicity. Which goes back to the late 7th and the adoption of interchangeable parts. This is a pretty old concept. Alive and well. Incredibly simple. Now I'm not saying throw it away. I used to think throw it away. And I alienated some people by saying throw it away. So I don't, I'm not, I would say there's a place for that.
But there's also a place for question number two. Question number two is How many ways are there for this quality characteristic to meet its requirement? And there I say the answer is infinite. Because if you look at you know decimal places, you know there's an infinite number of answers Between any min and any max, you can come up with an infinite number of [00:33:00] numbers that fit in.
One of the first times I said, infinite, 100 people in the room, and one guy says, infinite, and I said, okay, 463. But there's an infinite number. Again, if you take into account more and more decimal places, so that's called variation. So question one is not about variation. Question one is yes or no. Black and white thinking question two is looking at variation in good and my first exposure to this variation and good was the engineer at the jam plant saying you ought to look at it as shades of gray.
And so, so when he said so, so little did I know that he was introducing me to shades that shades of gray thinking is part of how to get your looks at quality. And so. But the next thing is, where I think people get very confused, is in terms of this variability reduction thing. And even in Larry Sullivan's article, what's on page two [00:34:00] is reduced variability, and this is where people get lost.
So the way I've been explaining it recently is there's precision and accuracy in the world of measurement. So precision is getting the same value each time. So I was, I donate blood platelets every other week, and I have a number of friends who do that. And, and if you're in need of blood platelets, you need people that donate them, which is, which is not an easy thing to do.
You're in the, on the machine, having blood taken out of one arm and into the other for two hours, and not everybody has that time. So anyway, so I donate, and one of the things they do before they measure your pulse has to be within a set of requirements. Your blood pressure has to meet requirements and your hemoglobin has to be within requirements.
And I have a couple of years ago, I failed several times in a row because my hemoglobin didn't meet requirements. So when they measured it last time, they take a drop of blood, put it in, in, in, in this plastic part, put it into the machine. So I've done [00:35:00] this hundreds of times. This time, she took it out of the machine.
She's going to throw it away. I said, no, I said, put it back in the machine. So she puts it back in the machine. And the reading was, went from 13. 7 to 13. 6. So she takes it out. She's like, can I throw it away now? I said, no, put it back in the machine. Next time it's the 13. 6. Well, that, that getting results that are very, very close together is precision.
But depending on the last time that machine was calibrated, it may be precise, but not accurate. So. On a dartboard, accuracy is getting on the bullseye every single time that's accuracy. You know, being three inches to the right of the bullseye every single time is precision. And so if the requirements are to be on the dartboard, having a cluster close together, not on target, is precision.
So if [00:36:00] you go off and look at people that are chasing, in the world of quality, variability reduction, You will hear them say piece to piece consistency is the name of the game in quality. That is about standard deviation. Standard deviation is the average variation from each other. That's what Six Sigma Equality people are doing.
That's what Larry Sullivan was doing. So this measure called Process Capability Index, C, is measuring precision. You get high values of CPK by having a small standard deviation in comparison to how far you are. From the nearest spec. Okay. Did that explanation. Yeah. Yeah. Well, I mean,
John Willis: in the go no go scenario, right?
There's sort of the outer and inner spec. And so you're saying that's the standard deviation from either of those 2 specs,
Bill Bellows: right? Well, what you're doing is so back to the dartboard. You're looking for what's the [00:37:00] average amount of variation. From these darts, from each other, right? That's, that's sick. And then now what you do in the CDP Kena calculation is take the average value of that cluster and you look at the distance to the nearest, the nearest requirement.
Right. I mean, so for a dart board, you've got requirements left, right, top, bottom. Right. But let's just say you're looking at the requirements left and right. So you say, I've got a really tight cluster and now you take. What's the distance from the average to the nearest spec and that distance to the nearest spec is on the top of the equation for process capability index.
And then I divide that by the, you know, three times the standard deviation. And, and what I'm trying to explain is you can be incredibly precise a little bit away from the edge, the requirement and have a great CPK. But nowhere in that conversation. Are we talking about the bullseye? [00:38:00] What Dr. Taguchi is talking about.
Is the bullseye and what is the amount of variation of each dart throw from the bullseye. And now you get into why does that matter? So, so 1st, understand that in the world of 6 sigma quality, all that variability reduction stuff is about precision
John Willis: to to shoot though, to is that what you're saying? So, if you don't take into account Taguchi's work to even sort of sure, it's control charts, right?
You're saying that's about precision? Yeah.
Bill Bellows: Right? Because we're just, well, I would say, yeah, yeah, yeah. Good point. And I'm okay
John Willis: with that because that helps me better understand what the difference is between what Taguchi' saying. There's a line there
Bill Bellows: and let's measure, well, let's go back to Schuert.
Schuert's work, my interpretation, Schuert's work is how predictable, how stable are we in getting that cluster? And And I would say Schubert's work was not so much [00:39:00] about improving precision, but just saying that for any level of precision, once the process is in control, then that next dart throw is going to be somewhere within the control limits.
But the control numbers could be, you know, very narrow, very wide, but
John Willis: I guess what I'm thinking then is what you're saying, though, it still falls prey to the same problem. It is. Yes. I mean, you're right. You're identifying a process and all the stuff that that, you know, about control charge and choose work is the, you know, the common cause special all that.
Right? But yes, but at the end of the day, if it's all about precision, Like, like the blood, the thing that mounted your hemoglobin, right? Like, that could be showing me this incredible, you know, chart of, look how good we're doing. We're staying, like, we're variable against this sort of the mean, and it's within one standard deviation or two standard deviations, or even three, depending on how you're counting.
[00:40:00] But it could be all wrong. Because, well,
Bill Bellows: yeah, well, well, when it comes to a measurement when, when it's relative to the hemoglobin, we may be very precise, but depending on the last time that thing was calibrated. That 13. 7 could actually be 11. 7, which would actually be. Yeah. Yeah. I mean, my, my interest. And that in the hemoglobin is I've had times when it measures 12.
3 and if it's 12. 3 you can't donate because it's not 13 and and so imagine I, you know, I get in traffic drive to this thing for 45 minutes and it's 12. 3 well now another person comes into the room to measure it. So they record that value. Now, another person has to come in and measure it, and if it's still under 13, they say goodbye, and they drive home, and the sad thing there is, that spot I reserved now can't be used, so not only can I not donate, but no one can donate, so, so, but, but [00:41:00] I've had the second reading turn out to be 13.
Right, right. Yeah, and that So I've seen So part of the reason I brought that up is that, and I, and I know the 12 3 is, is, can become 13, but now I'm, I am, and I don't know how much of that was the device versus the measurement versus the drop of blood, but I am, I am, for some other reasons, I'm more interested in what is the level of precision of that device.
I don't care about the accuracy. I just figured they're, they're worried about that. But for our point. I think what you're saying is, in Shewart's world, it was about the process being in control, which means, you know, not that it's very precise, it's, it's at the level, the amount of variation is consistent.
So that's not about improving precision, it's just saying, because all those darts can be thrown and they You know, some big cluster all over the place. And what I was sure I was talking about is, is it predictable and [00:42:00] stable? And then, and then simultaneously, I'm sure what was, you know, easily on his mind is, does it meet requirements?
Yeah. And the better, and if you're in control and it meets requirements, then, hey, that's, that's question number. I guess
John Willis: that's the interesting question, right? So like in the dart example, let's say I have a team and we have three people and, and we're, you know, we're constantly getting beat, but we're, we're, we're, we're watching all our darts and they're all falling out in the sort of second and third layer.
And from a control chart perspective, we are, that's our process. That's what we're good. And all of a sudden we bring in a ringer and now all of a sudden this ringer is hitting the right around the bullseye, hitting the bullseye, right? And, and so in the first example, we're not taking into account what you're saying is, you know, the, like, it's not about reducing variability.
It's, it's getting to accuracy or at least understanding the accuracy. Well, it's
Bill Bellows: a fascinating conversation. In the world of darts, At least I'm not confusing you. No, no, no, no, no. But [00:43:00] in the world of darts, why is accuracy important? Because there's more points for being on the bull's eye. That's right. Yeah.
So in the world of darts, first thing I want to find out is. You know, I want to improve my precision. I want to, I'd be happy to be on the dartboard, very precise, and boy, if I can do that, and then I'm thinking, well, the next thing I want to do is now, how can I, you know, now you're thinking, I want to throw it the same way, but maybe I need to step a foot to the left, and then I, and that will, so I'm just going to slide over.
And I'm going to maintain the precision, but I just need to adjust, I need to take that distribution and somehow get it onto the bullseye because then I get more points in this game and I'm, I'm, and I want to beat John, right? So, so in the world of darts, I could see people focusing on precision. And then I could see them, you know, take a half a step to the left, maybe they think I need to back up a [00:44:00] little bit, but I want to keep I want to keep that throat the same.
I just want to get. Well, I think
John Willis: you, you talked about in 1 of your podcasts about a good example, the white beads, right? Precision is like, getting all the getting all the red beads, which, by the way, never happens, right? There'll always be red beads in the way scenario, but let's assume that you get to a world where you reduce the variation to almost.
Nothing of you only
Bill Bellows: get of the white, white beads of the red beads. Yeah. Reduce
John Willis: the red beads. I mean, you're all white beads, basically. Yeah. That's what I meant. Right. So, and, but, but then the, the, that sort of a precision thing, but then the thing I think you brought up is, okay, what about the sort of the quality?
The, you know, so they all
Bill Bellows: meet. No, not, not, not quite. Not quite. Yeah. Okay. If we're talking about red beads and white beads relative to Dr. Deming's red bead experiment. Right. Right. Yeah. And the ambition is, you put the paddle into the bowl, you get a number of, you know, there's 50 indents in there, [00:45:00] and let's say, so you've got a paddle which is like the size of a 3x5 card, and in there, there is 50 holes in a five by 10 grid in these beads, which have a diameter of, let's say, an eighth of an inch and you've got 4, 000 beads in there.
Most people probably
John Willis: know that, know the red bead game. So,
Bill Bellows: okay. But in the world of red beads and white beads, precision and accuracy don't apply. Well, I would say this. Precision,
John Willis: how do I reduce the variability to get to, if you could, in the red bead game you really can't because it's limited, but let's say you could, through suppliers or whatever, get down to batches of all white beads, no red beads, that's still a precision thing because there might be another vendor that makes shinier beads or beads that are, and I think there is an accuracy
Bill Bellows: discussion.
But here's, but here's the difference relative to this example. Okay. So in the one case we've got red beads and [00:46:00] white beads. Red beads and white beads. The red beads are the defects, the white beads are the good beads, right? Right. In terms of the dartboard, a red bead is a dart that doesn't get on to the dartboard.
Because, and what we talked about in the dartboard example is, is the requirement is to be on the dartboard, not on the wall. If you're an Olympic
John Willis: dart beating, if you're an Olympic dart throwing team, A red bead is not getting within the inner circle somewhere.
Bill Bellows: No, no. In, in the world of the red bees and the white beads is black and white.
They're, they're black, they're red or they're white. In the world of, of the dartboard we are if, if, if all the beads. Well, and in that world, being close to the bullseye is significant, right? Yeah, yeah. I mean it, it's, that's the . Okay. But that, but that dis but that distance from. The distance from the dart to the bullseye is, is variable.
Every time you throw it, it's a different distance. That's shades of gray thinking. [00:47:00] The red beads and the white beads is blocking, is blocking white beads. But
John Willis: what I'm saying is you had mentioned in some of the podcasts that the way you get into shades of gray thinking with the white beads is, okay, now you've solved the sort of defect problem, but now you might want to make shinier beads or more quality beads.
Bill Bellows: I did, but let me just say that where I came from. And that is don't tie this together is I said, one of the big lessons learned in the red bead experiment is that the red beads are not caused by the workers. The defects are not caused by the workers separately. They're caused by the system that includes the beads.
So don't blame the workers blame the system. And, and when I explained this commonly. That becomes the big aha for people getting exposed to Deming's work is, you know, the red, you know, first of all, there's, there's every time you put the paddle in, you get a different number of red beads and try it again, try it again, try it again.
And then you get a pattern that you can talk, put that data on a control [00:48:00] chart, number of red beads goes up and down within some set of control limits, which you can calculate. And so what I've done when I, when I worked for the Deming Institute, what I introduced. Was they so that the instructor would leave them on a path to don't look at two data points at a spreadsheet step back.
Look at the look at the data on a on a run chart converted to a rolled into a into a control chart. And then I would get up and say, you know, to the audience of 50, 60, 70 people and say, so we know the red beads are caused by the system, not the workers taken separately. But is your understanding then.
That what Dr Deming is saying is we need to eliminate the red beads everywhere in the system, go to our supplier, stop buying. Maybe the red beads are coming from the supplier and we don't want it. We don't want them. And we don't want them in our system. Maybe we have to work around the organization to figure out how to get rid of the red bead.
And then I said. Is the ambition is what Dr Deming is [00:49:00] saying. For continuous improvement is to strive to have fewer and fewer and fewer and fewer red beads until there's none. But. If they are none, how do we improve? So if Dr. Deming said continuously improve, but we get to no red beads, are we done? And then people would say, well, we can make them faster.
We can make them cheaper. I said, fine. I said, but can we improve the quality of the white beads? If all the beads, white beads are white, and we make them faster and cheaper, which is faster. And cheaper, but that's not better. Is there a better? Is there a better white bead? That's for people to get stumped.
And and so my ambition was to create that awareness, that awareness of that is, is what's ingrained with me from all those years with Dr. Tsuguchi, that variation [00:50:00] within the requirements is, could be very significant. I guess that's what I was,
John Willis: I guess I'm struggling to get to explain. That's what I'm trying to say is I think it is that, that, that you are, you know, maybe it's not an accuracy thing, but in the sort of the dark board example, but to me, when I heard that.
That to me, that was the difference between understanding variation in Taguchi's work is that
Bill Bellows: yes, you've reduced
John Willis: the variation to and, and we both know you can never reduce it to zero, but, but, but let's say you've gotten it to a system level where you've gotten at a system level, you've like one in every how many red beads come in and let's say, maybe there is a world and some of the universe where it can always be zero, but Not in this world, but, but even then, then to me, that's the difference between your dartboard example of precision versus, can we make beads of better quality now that we've [00:51:00] gotten all the defects out?
And that to me sounds like the
Bill Bellows: accuracy. Well, I would say this.
I get, I can see that if we get it to 100 percent white beat, so there could be, you know, 70 percent white beads, 80 percent white beads. Now we can get to 100 percent white beads and it's always 100. So that's precise. And you might even argue that's accurate because you could say, that's the goal. I want to be, I want that the ideal because accuracy is about the ideal value.
Precision is about being consistent to each other. And so, yeah, it may be there's variation in the number of whites, and I'm looking for less and less variation in white until they're 100 percent white, and now I, now I have accuracy and precision, and trust me, there are people that think that's the, that's accuracy and precision, but that's not accuracy and precision that Taguchi's talk could be.[00:52:00]
But that is, now that we've gone through this, that is accuracy and precision. But what Taguchi is doing is taking it to a new level. Taguchi is saying that the way to improve the quality of the white beads, when they're all white, is to realize that they're not the same shade of white. They aren't the same diameter.
They aren't the same white weight, weight. They may not have the same, you know, I mean, they may not be exactly spheres. And, and so now we get into this very You know, what Taguchi was trying to expose relative to quality is question two, not question one. Question one is, white beads or red beads, white beads or red beads.
What Taguchi' talking about is, [00:53:00] is there's variation in good. And so I liken it to the difference between saying, John, does the car have gas? And you say, yes. John, how much gas is in the car? So they may be white, but there's degrees of white if we treat white as good and that very simple model of red beads and white beads.
We're saying red is bad. White is good. And that's that's question. Number 1 doesn't meet requirements. Yes or no. What's Taguchi is talking about? Is there's variation in good. And now, if all the darts are on the dart board, then they're all white beads. They're all good. If we have a cluster at the. You know, at, at the third base location, or, you know, the number three position on the dartboard, and if they're all incredibly close to one another, that's precision.
Darts, I'm not saying [00:54:00] all dartboard, all dart games, but there are dart games where being on the bullseye. Is worth more and that's what Taguchi is talking about relative to accuracy, not the 100 percent white beats. I think so.
John Willis: Let me say it 1 more time because I think it to me. It sounds it is the same thing.
Just in words. And we say the dartboard if we say that good is on the board and precision is a cluster of a bunch of ones that don't vary much from themselves. Accuracy is. Like, so we look at that and we say, well, this is, this is good, right? It's, we've reduced it. In fact, we're even getting that cluster tighter and tighter and tighter, but it's on third base.
Right? So I, I, I, unless I'm missing, I see that it's the same thing as the white boards. I mean, all the white bees are equivalent to getting the, all the darts on the board to be good. And then, but then the Taguchi is that sort of dissimilar to [00:55:00] that's. Yes. Okay, that's good. Now, let's look at good in the white beads and what's the variation of the shape, the size, the color, the, you know, the, so to me, it seems like the same.
It seems like a precision accuracy, even in the white in the way
Bill Bellows: you've it is. So, yeah, it is about precision and accuracy, but it's a precision and accuracy from a black and white perspective to precision and actually in a shades of gray perspective.
John Willis: Yeah, I guess at that point, I was just looking at as sort of an accuracy discussion, but yeah, I guess it's precision.
It's a, it's within good. Now you're actually looking at the precision and accuracy within good.